Introduction
Dashboards and reports are the lingua franca in the world of business. Simple as they may seem, behind each KPI dashboard are data analysts who are responsible for keeping dashboards working, accurate, and fresh.
For small teams with a handful of data analysts, building dashboards is easy. They’re familiar with every dataset and it doesn’t take long to write the queries they need. But for larger organizations, the amount of data dwarfs any one person or team’s ability to understand all of it.
Whenever there’s a business question, analysts can save the day by putting together their dashboards or analysis. But one business question often leads to another, and reaching 50 or 100 dashboards will happen in no time (ask anyone who knows — if anything, this is an understatement). What if two different people have the same business questions to answer? Do they know which report to refer to? Will they look at the same report? For analysts and those who are more familiar with the BI tool, composing a new metric from scratch will often take less time than looking for an existing metric. This creates inconsistencies and can even lead to inaccurate results and misguided decisions.
How can we facilitate better visibility and understanding?
Data Discovery Platforms
So far, the best solution to bridge this knowledge gap has been to periodically clean up dashboards and documentation. However, the underlying data will keep changing for any number of reasons:
- Your organization releases a new product and starts collecting different types of data.
- You start using new software to keep track of business operations, which you might want to join with your current data.
- You develop a new set of business KPIs to keep track or change the way you calculate metrics as the business evolves.
Because data is always changing, documentation requires constant upkeep and BI dashboards can quickly become stale or even wrong. It becomes even harder as analysts change roles or leave the organization — when a process relies on one person’s built-up knowledge it can easily break down if that person’s situation changes.
There is a problem with information asymmetry between data analysts and data consumers (the business/product teams relying on these dashboards). Namely, the context of data used within an organization remains siloed as tribal knowledge in the data team. As I detailed in my previous blog post, hyper-scale companies like Netflix, Spotify, and Airbnb have begun consolidating metadata and its documentation into one place called a data discovery platform.
These data discovery platforms are all a centralized place for anyone in the company to find the data they’re looking for, see who else is using it, where it’s being used, and share their knowledge about it. By plugging BI tools into these platforms and collecting metadata, both data teams and data consumers can greatly benefit from having the end-to-end visibility and context around how the data is being defined and used inside the company. Here are three ways that BI integrations have been the most beneficial to data-driven organizations.
1. Understand Upstream Dependencies & Downstream Impacts
When BI tools are connected with data lineage, the data transformation flow is visible from start to finish. With data lineage, data producers (usually software engineers or data engineers) are aware of which reports or dashboards may break from a field name change or table deprecation. By understanding the potential downstream impact, data producers can coordinate with the data consumers (the BI team or data analysts) to ensure a smooth transition when changes need to be made. That way, your engineering team might think twice before deprecating a column, or deleting a source table.
When data lineage is integrated with BI tools, data analysts can understand all the upstream dependencies of their reports and dashboards. When the report numbers look abnormal, they can traverse the lineage tree to look up the dependency tables and their freshness. Without data lineage, finding out exactly where in the data flow has resulted in a wrong output is like black box testing — a lot of disjointed poking around tables, logs, and SQL queries. Data lineage makes it much easier to isolate and correct the issue.
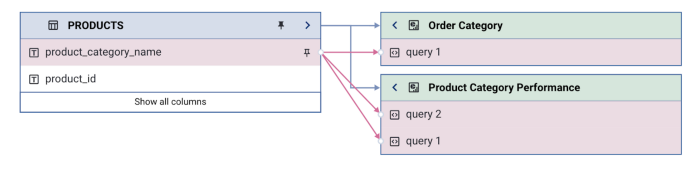
2. Declutter Old Models & Dashboards
Serious data question: how do companies deal with dashboard rot? Everywhere I've worked, 95% of all dashboards are half-broken or not trustworthy because no one has touched them for 6+ months. Are dashboards inherently ephemeral?
Any company that has had a BI tool for more than a year will deal with the dashboard clutter problem. Ad-hoc analysis, quarterly reports, and even core dashboards get outdated or change to a new version over time. The problem is, old dashboards usually don’t get deleted. No one wants to delete a dashboard in the shared folder because someone might be using it. This creates a long tail of clutter and inactive reports that people may poke around in, but they won’t be sure if the data is reliable or relevant. Navigating BI tools becomes its own tribal knowledge task and, it ends up being best to ask others to send you a specific link to open. What could be worse is that there may be someone relying on an outdated dashboard for their day-to-day operations.
This often happens because dashboard metadata and its freshness isn’t tracked automatically. Connecting dashboard metadata along with its operational metrics like the last successful report run, last edited time, and top users can give visibility into the health of the dashboard. By comparing usage data along with operational metrics, outdated data models can easily be identified and cleaned out. It also becomes clear to data consumers if the dashboard isn’t being maintained or used by others anymore.
3. Empower Self-Service Analytic
Modern BI tools give non-technical users a way to manipulate data without having to learn SQL. They make it incredibly easy to drill down on data on an ad-hoc basis. But even with the easiest tools, building a dashboard is still hard for many data consumers because they do not have the background or context of the data. For example, when there are 20 dimension fields, what are the most common ways to slice the data? If there are fields with similar names (e.g., status field vs. active_status field) which one is the right dimension to use? By including the field level usage information, data discovery platforms can provide guidance to data consumers on how to use the data.
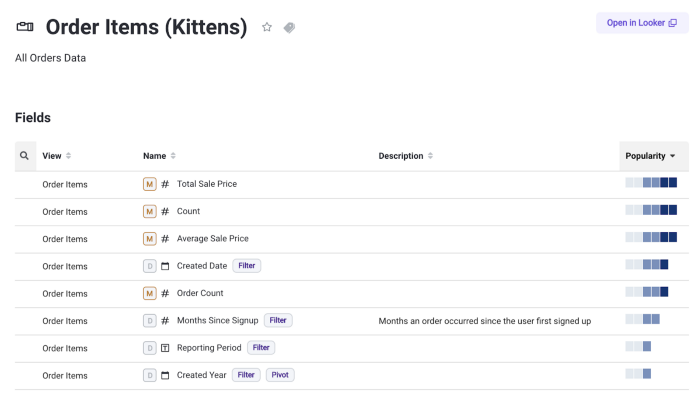
Many times, data consumers who want to self-service their own analytics needs end up in a sea of tables and fields. It’s discouraging to try understanding how the data is represented without having any documentation or guidance. Data discovery brings that context to the forefront, like in Figure 3, which shows the popularity of each field or which dimensions and measures are being used as a filter or pivot in other dashboards. The added context can guide new data analysts or consumers as they get acclimated with the data, empowering them to explore and run their own analysis.
Bring Full Visibility of Your Data with Data Discovery
In time, data discovery platforms will grow to become the de facto tool for employees to find and learn about data that’s used throughout the organization. As a centralized metadata hub, they embrace how data changes over time and provide analysis and recommendations for analysts, data scientists, and business users to quickly find what they’re looking for.
At Select Star, we believe BI integration is a core part of the data discovery platform. We’ve built deep integrations and metadata analysis behind the modern data tools including Snowflake, BigQuery, Tableau, Looker, and Mode, to provide end-to-end data lineage and operational usage metrics. We’re helping fast-growing startups to fortune 500 companies to build a single source of truth documentation & governance on their data. If you’re looking to understand your data and provide better self-service analytics experience for everyone in your company, we’d love to hear from you.