Establishing clear data ownership is essential to making data catalogs useful, building data literacy across teams, and accelerating data-driven decision making. Without defined ownership, even the best cataloging tools struggle to stay up to date. Documentation becomes stale, trust erodes, and data assets lose their value as a strategic resource.
At Select Star, we’ve worked with many organizations to improve how data is documented, accessed, and understood. A consistent takeaway from these implementations is that success depends on assigning the right people to take responsibility for data—both at a technical and business level. Ownership and stewardship are not optional roles; they are the backbone of discoverability and collaboration across the modern data stack.
This post explains how to build a scalable framework for data ownership. We’ll cover how to define data domains, assign appropriate owners and stewards, and establish sustainable practices that ensure data remains trustworthy and useful long after it’s created.
Table of Contents
- Understanding and Defining Data Domains
- Assigning Data Stewards and Domain Owners
- Training Data Stewards to Succeed in Ownership Roles
- Building Sustainable Data Ownership Workflows
- Fostering Cross-Functional Collaboration in Data Ownership
- Best Practices for Effective Data Ownership
- Strengthening Your Data Strategy Through Ownership
Understanding and Defining Data Domains

Before assigning ownership, organizations need to define their data domains. A data domain refers to a logical grouping of related datasets that typically map to a business function, such as sales, marketing, finance, or product.
Data domains serve two purposes. First, they provide a clear scope for ownership, avoiding overlaps and ambiguity. Second, they align data responsibility with business knowledge. For example, the marketing domain may include data pipelines related to campaigns, lead scoring, and web analytics, while the finance domain covers revenue reports, expense tracking, and forecasting models.
Successful domain definitions are usually based on existing organizational structures. This alignment ensures that people closest to the data also hold responsibility for its accuracy and usefulness. Companies often use their organizational chart and existing reporting lines as a starting point when mapping data domains, which helps keep ownership intuitive and operationally practical.
Assigning Data Stewards and Domain Owners
Once domains are clearly defined, the next step is identifying who should be responsible. This typically involves distinguishing between technical ownership and business ownership—two complementary but distinct responsibilities.
Technical owners are usually analytics engineers, data engineers, or platform leads. They ensure the data pipelines are reliable, datasets are properly structured, and metadata is complete. These owners often take on the data steward role, handling documentation, coordinating schema changes, and resolving operational issues. They are closest to the systems and infrastructure that support data access and usability.
Business owners, by contrast, are responsible for ensuring that the data aligns with business goals and decision-making needs. They might be heads of departments or domain experts who understand the context and interpretation of the data. Business owners validate that the metrics, definitions, and usage of data are correct from a business perspective and help guide prioritization for new data initiatives or improvements.
Each data domain should have both a technical owner and a business owner. For example, in a sales domain, the technical owner might manage the ETL processes populating Salesforce data into the warehouse, while the business owner ensures that definitions like "closed deal" or "lead conversion" are consistent and understood across reporting layers.
This dual model of ownership ensures that both the infrastructure and the context of the data are managed effectively. It also facilitates better collaboration between technical and non-technical stakeholders, ultimately leading to higher trust and better use of data across the organization.
Training Data Stewards to Succeed in Ownership Roles
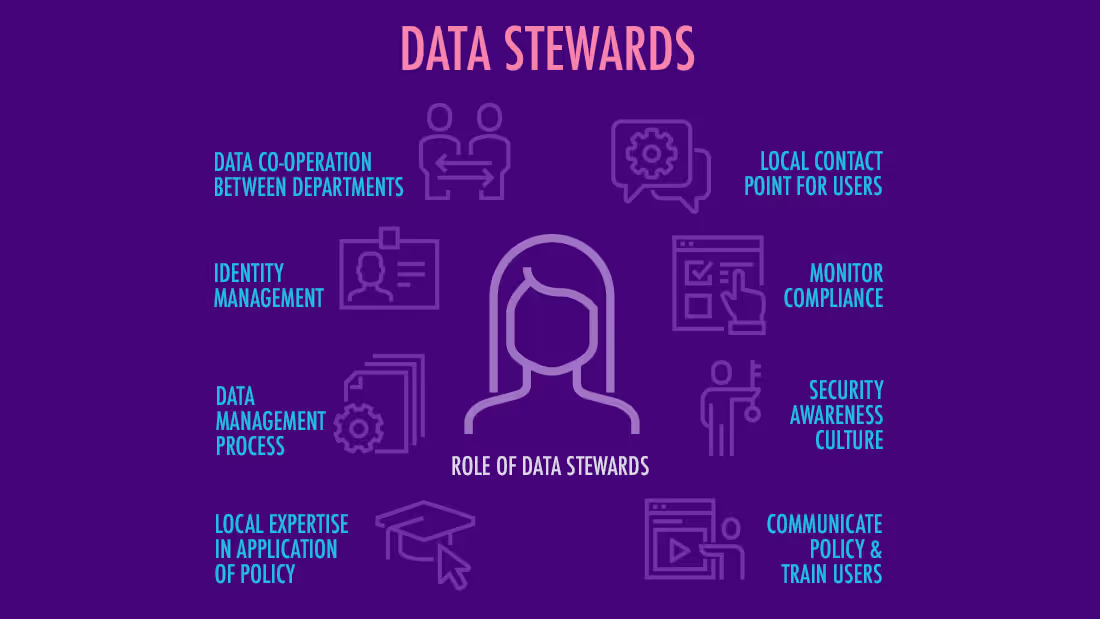
Assigning roles is only the beginning. Many organizations struggle because data stewards are given responsibilities without the tools, training, or time to succeed.
Effective training programs should include three core areas:
- System knowledge: Stewards need to develop deep familiarity with the tools that support data discovery and understanding—especially the data catalog. This includes learning how to document datasets, interpret lineage graphs, apply metadata tags, and monitor asset usage within the catalog. They should also understand how the catalog integrates with other parts of the data stack, such as the data warehouse, BI tools, and transformation pipelines.
- Governance policies: They must be familiar with internal data governance protocols, such as naming conventions, access controls, documentation standards, and quality metrics.
- Workflow integration: Stewards should be trained to incorporate stewardship responsibilities into their daily routines, ensuring tasks like documentation and quality checks become part of their standard operational flow.
One approach is to create a steward onboarding guide that outlines expectations and checklists for key activities. Many companies formalize these into internal documentation platforms, often with embedded dashboards showing steward activity—like the number of tables documented or lineage issues resolved in the past month.
Organizations that automate monitoring of stale datasets and missing documentation empower stewards to act proactively. This helps turn governance into an active rather than reactive process and embeds it into the rhythm of everyday work.
Building Sustainable Data Ownership Workflows
Without ongoing maintenance, even the best stewardship programs degrade over time. It’s essential to build regular, repeatable processes that reinforce data ownership.
This includes automated alerts for stale tables, schema changes, or unexpected drops in data freshness. Many teams set up weekly or bi-weekly "data quality standups" where stewards review key metrics and align on upcoming changes. These meetings are especially valuable in fast-growing companies where the data landscape evolves rapidly.
Another best practice is using metadata activity logs to monitor steward engagement. For instance, if a table hasn’t been reviewed or updated in over 90 days, that might trigger a task in your ticketing system or workflow tool. Some organizations pair this with tags or status labels in the data catalog to indicate whether a dataset is actively maintained, under review, or deprecated.
Additionally, domain owners should conduct quarterly reviews to assess stewardship effectiveness. This includes checking whether documentation coverage has improved, how many issues have been resolved, and whether data consumers report higher confidence in the data. These metrics help demonstrate the ROI of stewardship to business leaders.
Fostering Cross-Functional Collaboration in Data Ownership
Data doesn’t exist in silos. Ownership structures need to accommodate cross-functional dependencies. For example, a marketing dashboard might rely on product usage data, which falls under the product domain. In such cases, stewardship responsibility should be clearly delineated through data lineage tools and mutual agreements on SLAs.

Data lineage can help map upstream and downstream dependencies, making it easier for stewards to identify when changes to one domain may affect another. Documenting these dependencies helps reduce blame-shifting and encourages shared responsibility for data reliability.
When implemented well, these visibility layers enable organizations to trace data issues to their source quickly and assign remediation tasks efficiently. This increases accountability and shortens resolution times.
Best Practices for Effective Data Ownership
To make data ownership truly effective, organizations need to treat it as an evolving discipline rather than a one-time setup. That means integrating stewardship into performance reviews, celebrating teams that improve documentation coverage, and continuously iterating on domain boundaries as the business grows.
One often overlooked practice is including data ownership expectations in job descriptions for analytics roles. This sets the tone early and helps reinforce stewardship as part of the culture. Similarly, some companies provide incentives or recognition for stewards who maintain high data quality, whether through internal shoutouts or contribution leaderboards.
From a tooling perspective, automating the detection of data issues and tracking steward actions helps avoid burnout and ensures teams focus their efforts where they matter most. Over time, this reduces the operational load on central data teams by distributing responsibility across the organization.
Strengthening Your Data Strategy Through Ownership
Assigning and maintaining data ownership is critical for any organization aiming to scale its data infrastructure responsibly. By defining clear data domains, empowering stewards with the right tools and training, and establishing ongoing maintenance workflows, companies can ensure that data remains reliable, discoverable, and trustworthy.
More importantly, effective data ownership structures lead to better business outcomes. Decisions are made faster, issues are resolved quicker, and teams feel confident using data to drive strategy. As the data landscape becomes more complex, these practices will only grow in importance. Organizations that invest early in data stewardship will be the ones best equipped to adapt and thrive.