Managing Snowflake costs effectively has become a critical concern for organizations as data volumes grow and usage patterns evolve. Understanding data usage patterns is crucial for optimizing Snowflake costs and improving overall data governance. Shinji Kim, founder and CEO of Select Star, recently presented at a Snowflake User Group meeting on Snowflake Cost Optimization. This post recaps the strategies presented for leveraging data usage context to drive Snowflake cost optimization initiatives.
Select Star, an intelligent metadata platform, provides insights into these aspects by analyzing query patterns, joins, and filter conditions. This information is derived from Snowflake's account usage and access history views, offering a comprehensive understanding of data utilization.
Table of Contents
β
- Understanding Usage Context in Snowflake
- Strategies for Snowflake Cost Optimization
- Real-World Cost Optimization Success Stories
- Implementing Usage-Based Cost Optimization
- Future Trends in Snowflake Cost Optimization
Understanding Usage Context in Snowflake
.png)
Usage context refers to the comprehensive understanding of how data is being accessed, transformed, and consumed within your Snowflake environment. Effective cost optimization begins with a comprehensive understanding of how your data is being used. In Snowflake, usage context encompasses four key aspects:
- Where did the data come from?
- Who is using the data?
- Where does the data get used?
- What other datasets can be used with this data?
By analyzing query patterns, including select statements, create/insert/update operations, and join conditions, organizations can gain valuable insights into their data ecosystem. This information is crucial for identifying opportunities to optimize costs and streamline data management processes.
Strategies for Snowflake Cost Optimization
Leveraging usage context, organizations can implement two primary strategies for Snowflake cost optimization: deprecating unused data and remodeling expensive data pipelines.
1. Deprecating Unused Data
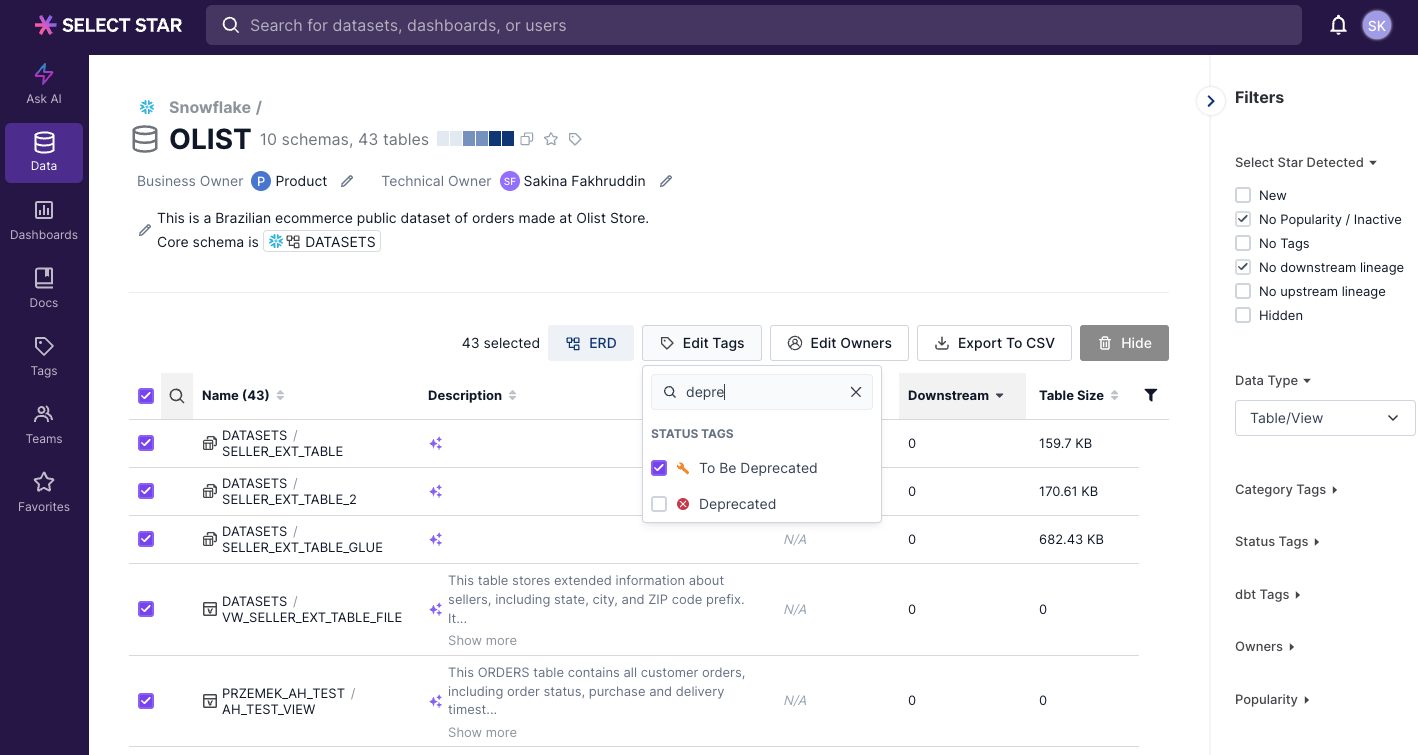
Identifying and removing unused data is a straightforward yet effective way to reduce costs. Organizations should consider several key areas for optimization. These include tables that have been inactive for the past 90 days, outdated data that continues to be ingested, ETL pipelines lacking downstream dependencies, unused dashboards connected to Snowflake, and objects without any downstream usage. By addressing these areas systematically, companies can achieve significant cost savings. Not only do these efforts reduce storage costs, but they also eliminate the associated compute costs that arise from processing unnecessary data. This dual benefit makes data cleanup a crucial component of any comprehensive Snowflake cost optimization strategy.
2. Remodeling Expensive Data Pipelines
Analyzing and optimizing costly queries and data pipelines can lead to substantial savings. Organizations should focus on several key areas to maximize efficiency. First, examine queries that access tables with numerous columns, as these can be particularly resource-intensive. Next, evaluate the utilization of raw versus refined or aggregated tables, ensuring data is accessed at the appropriate level of granularity. Consider implementing incremental data refresh processes instead of full refreshes where possible, and review refresh schedules to strike a balance between data freshness and cost. Finally, optimize join operations to reduce query complexity and improve performance. By addressing these areas systematically, companies can significantly reduce their Snowflake costs while maintaining data integrity and accessibility.
Real-World Cost Optimization Success Stories
Several organizations have successfully implemented these strategies with Select Star to achieve substantial Snowflake cost savings.Β
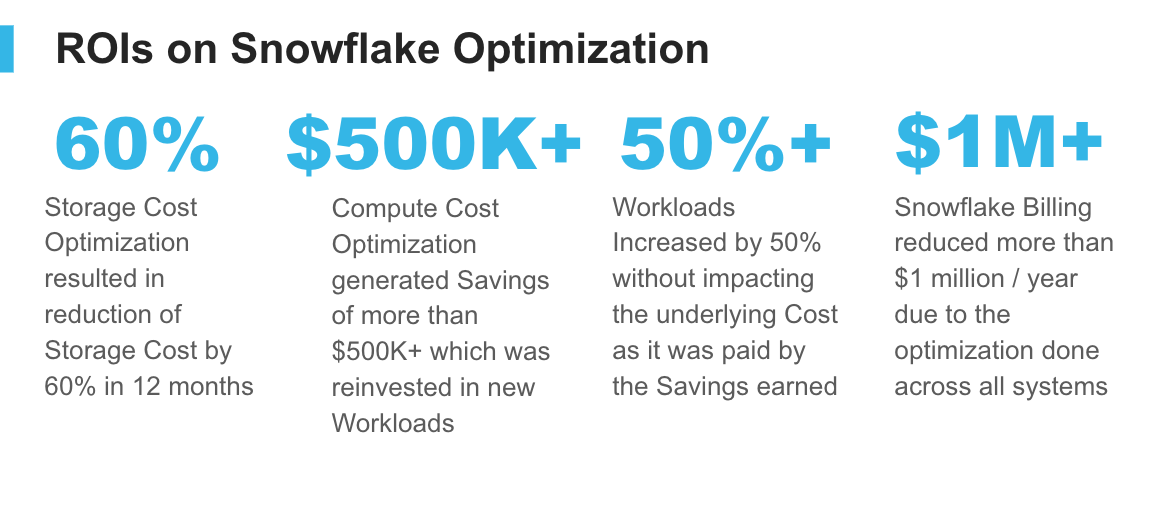
Pitney Bowes, a Fortune 500 company, saved over a million dollars annually by leveraging usage information to reduce storage costs and deprecate unnecessary jobs. They utilized Select Star to identify underutilized BI dashboards and optimize their most expensive instances.
A fintech company focused on migrating their ETL pipelines, starting with an analysis of Tableau workbook usage. By working backwards to identify utilized source tables, they reduced their data engineering workload by 38%.
Faire, during their migration from Redshift to Snowflake, remodeled their data pipelines based on column-level usage patterns. By understanding which columns were frequently utilized, joined, or selected together, they created a new, optimized data model that significantly reduced their Snowflake operational costs.
Implementing Usage-Based Cost Optimization
To implement these strategies effectively, consider the following steps:
- Regularly audit data usage: Implement tools or processes to continuously monitor and analyze data utilization.
- Prioritize high-impact areas: Focus on deprecating unused leaf nodes and optimizing the most expensive queries first.
- Collaborate across teams: Engage with data consumers to ensure optimizations don't disrupt critical business processes.
- Implement gradual changes: Start with low-risk optimizations and progressively tackle more complex areas.
- Monitor and iterate: Continuously track the impact of your optimization efforts and adjust strategies as needed.
While usage-based cost optimization offers significant benefits, organizations should be aware of potential challenges. Ensuring proper governance and compliance when deprecating data is crucial, as is balancing cost savings with maintaining necessary historical data. Organizations must also manage the impact of data model changes on existing reports and analyses, which can be complex and time-consuming. Additionally, coordinating optimization efforts across multiple teams and stakeholders requires careful planning and communication to ensure alignment and prevent disruptions to critical business processes.
Future Trends in Snowflake Cost Optimization
As data ecosystems continue to evolve, we can expect to see advancements in cost optimization techniques:
- Integration of machine learning algorithms to predict and prevent cost overruns
- Automated optimization recommendations based on usage patterns and query performance
- Enhanced visibility into cross-platform data usage for multi-cloud environments
- Tighter integration between cost optimization tools and data governance frameworks
Cost optimization in Snowflake is an ongoing process that requires continuous monitoring and adjustment. By leveraging usage context and implementing targeted optimization strategies, organizations can significantly reduce their Snowflake expenses while improving overall data governance and efficiency. While Snowflake's interface can help identify expensive queries, deeper insights into usage patterns, dependencies, and optimization opportunities are crucial for making informed decisions about resource allocation and data model restructuring. To learn more about how you can understand your Snowflake usage for costs, explore Select Star's cost optimization offering.