Modern data governance is no longer just about regulatory compliance or locking down access. It has become a strategic function that helps organizations scale their data operations, improve collaboration, and increase confidence in analytics. As data volumes and complexity grow, governance needs to go beyond policy enforcement to actively support data discovery, clarity, and quality.
This evolution requires a shift in mindset—from isolated IT-led processes to shared, cross-functional responsibility. Modern data governance enables teams to work with data more effectively by embedding context, ownership, and trust directly into day-to-day workflows.
From aligning stakeholders around shared metrics to automating lineage and metadata management, we have helped organizations build governance into their day-to-day workflows. This article explores what modern data governance looks like, why it matters right now, and how to implement it in a way that delivers real operational impact.
Table of Contents
- What is Modern Data Governance?
- Why Modern Data Governance Matters: Beyond Compliance
- Key Principles and Best Practices of Modern Data Governance
- How to Get Started with Modern Data Governance
- Considerations and Pitfalls to Avoid
- Governance as a Strategic Enabler
What Is Modern Data Governance?
Modern data governance refers to a framework of policies, processes, tools, and roles that ensure data is accessible, trustworthy, secure, and used responsibly—not just for compliance, but for operational excellence and strategic decision-making.
Unlike traditional models that focus narrowly on control and restriction, modern data governance is:
- Proactive: anticipating and preventing data issues before they affect decisions or operations.
- Collaborative: involving data producers, stewards, and consumers across departments.
- Integrated: embedded in day-to-day workflows through data catalogs, lineage tools, and metadata management platforms.
- Business-aligned: driven by outcomes like customer experience, faster analytics, or product innovation—not just policy enforcement.
Why Modern Data Governance Matters: Beyond Compliance
1. Poor data governance stifles growth and efficiency
When data governance is reactive or fragmented, it leads to downstream chaos: duplicated metrics, conflicting reports, and decisions based on faulty data. Teams lose trust in the data and resort to siloed workarounds, increasing risk and inefficiency.
A 2022 Gartner survey revealed that over 60% of organizations struggle to trust their data for decision-making, despite significant investments in analytics and AI.
2. It’s essential for scaling self-service analytics
Modern data governance enables scalable self-service by providing context (metadata), clarity (definitions), and guardrails (access controls). When analysts can quickly find, understand, and trust the data they need, they spend less time cleaning and verifying, and more time generating value.
3. You can’t automate responsibly without it
With the rise of machine learning and AI-powered operations, data governance now plays a critical role in ensuring fairness, accountability, and ethical use of data. Governance policies need to guide model inputs, monitor drift, and manage sensitive attributes.
Key Principles and Best Practices of Modern Data Governance
1. Shift from enforcement to enablement
Traditional governance models focused on restriction—limiting who could access what. Modern governance flips this by aiming to enable access to trustworthy data, with appropriate context and controls.
Best practice: Define “certified” or “trusted” datasets in your data catalog (e.g., Select Star) and make them easy to discover via tagging, popularity rankings, or automated lineage insights.
Real-World Example: By leveraging Select Star, nib transformed their approach to data management, achieving a remarkable 90% increase in both efficiency and trust. This shift allowed them to move from reactive to proactive data management, empowering their team with the tools to work confidently with reliable, well-organized data.
2. Define ownership and accountability across the data lifecycle
Modern governance assigns clear roles for data ownership. This includes data stewards (who ensure quality and documentation), data product owners (who manage data as a product), and business stakeholders (who define what “good” data looks like).
Best practice: Use a RACI matrix to clarify responsibilities for data domains. This avoids finger-pointing when issues arise and encourages collaboration between data engineering and business teams.
3. Invest in metadata management and data lineage tools
Metadata is the backbone of governance. It provides critical context: where the data came from, how it’s transformed, and what it means. Automated lineage tools (like Select Star’s column-level lineage) let teams trace issues quickly and understand downstream impacts.
Best practice: Implement automated lineage tracking integrated with your data stack (dbt, Snowflake, Looker, etc.), and surface it directly in your data catalog for easy visibility.
Real-World Example By leveraging Select Star's automated column-level lineage, AlphaSense transformed their data warehouse operations, significantly reducing time-to-resolution for broken dashboards. After integrating Select Star with tools like dbt, BigQuery, and Looker, they identified inefficiencies, decluttered 66% of dashboards, and deprecated over 6,000 unused tables—turning hours-long root cause analyses into tasks completed in minutes.
4. Integrate governance into everyday tools and workflows
If governance only lives in a SharePoint document or buried policy PDF, it won’t be followed. Instead, surface governance context (like data descriptions, owners, and quality flags) where people work — in BI tools, notebooks, and data catalogs.
Best practice: Auto-sync documentation and ownership metadata from your catalog to tools like Looker or Mode, using integrations or APIs.
5. Measure and monitor governance impact
Treat governance like any product—it needs KPIs. Track metrics like data asset usage, documentation completeness, number of stale/unused assets, or time-to-resolution for incidents. Use these to guide improvements and show business value.
Best practice: Set quarterly OKRs around increasing trusted asset usage or reducing duplicative tables.

How to Get Started with Modern Data Governance
You don’t need a 12-month project plan to begin. Modern governance is best approached iteratively, starting with a few critical areas and expanding from there.
Step 1: Audit your current data landscape
Before fixing anything, understand what you have. This includes:
- Inventory of data sources, tables, reports
- Ownership and usage patterns
- Pain points (e.g., duplicated metrics, low trust, slow incident resolution)
Use automated discovery tools or catalogs to accelerate this. If you're unsure where to start, pick a key business area (like customer churn or financial reporting) and trace the data sources, models, and dashboards involved. This narrows scope and focuses governance efforts on high-impact areas.
Step 2: Define your governance goals and success metrics
Don’t just aim for “better governance.” Be specific:
- Do you want faster onboarding of analysts?
- Fewer incidents from broken dashboards?
- Improved data discoverability?
Translate those goals into measurable outcomes.
Step 3: Identify data domains and assign stewards
Choose 1–2 key data domains (e.g., customers, transactions) and assign data stewards to own documentation, naming conventions, and issue triage. These people are your governance champions.
Step 4: Implement a data catalog or governance platform
This is where metadata, ownership, lineage, and usage insights come together. A tool like Select Star provides visibility into your data assets, tracks lineage, and lets users tag, comment, and mark datasets as trusted.

Step 5: Start documenting, tagging, and cleaning as you go
Avoid waiting for a “documentation sprint.” Instead, build governance into daily workflows. When someone creates a new table or dashboard, encourage them to add a description, link upstream sources, and flag if it’s certified.
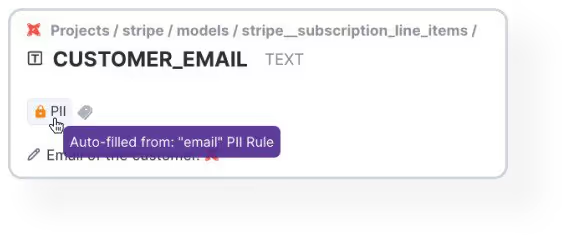
Step 6: Communicate and train
Modern governance is cultural, not just technical. Hold training sessions, create playbooks for data stewardship, and recognize teams who lead by example.
Considerations and Pitfalls to Avoid
Don’t over-engineer in the beginning
It’s tempting to roll out strict policies and tools all at once. But too much structure early on can create resistance. Start small and build momentum.
Avoid making governance someone else’s job
Governance isn’t just a data team responsibility—it’s a shared effort. Business teams, analysts, and product managers must all play a role in documenting, curating, and maintaining quality.
Governance ≠ Bureaucracy
Modern governance reduces friction by making data easier to find and trust. If your efforts are slowing people down, revisit your policies or tooling.
Real-World Example: Wallbox leveraged Select Star to establish a tiered system for data governance, categorizing key metrics and data assets based on their importance. This structure made it simple to trust and locate reliable data while removing redundancies, allowing Wallbox to improve consistency and clarity without creating roadblocks for their teams.
Governance as a Strategic Enabler
Modern data governance is not about saying “no” to data access—it’s about enabling the right people to find, understand, and use high-quality data with confidence. It’s a practice that supports—not constrains—data-driven innovation.
By shifting from reactive compliance to proactive enablement, and by embedding governance into daily workflows with the help of tools like Select Star, organizations can create a culture of trust, collaboration, and accountability around data. This leads not only to better decision-making, but also to greater agility, reduced risk, and more scalable analytics practices.
If you haven’t already started modernizing your governance approach, now is the time. Begin small, focus on impact, and grow iteratively. Your data—and your business—will thank you.